How we Deal with Small Data?
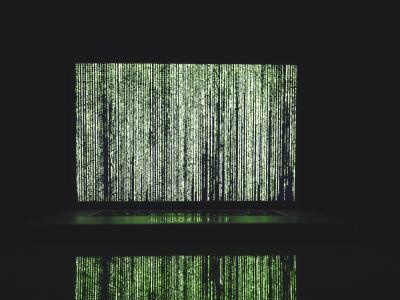
Abstract
Most commonly used statistical methods in analyzing and interpreting clinical trial data are based on a specific distribution of the data, such as normal, binomial and exponential models. The alternative is to choose nonparametric methods based on rank, but with the disadvantage that for small size samples, they may give erroneous results. In this article, we refer an accurate statistical method based on computational simulations in order to characterize and compare as efficiently as possible clinical trial studies having few values. The bootstrap technique is a statistical method that does not depend on any data distribution hypothesis and can be considered adequate to estimate some essential parameters in data analysis by different types of bootstrap confidence intervals. The effect-size statistics and its confidence intervals are also presented, being useful when we compare group means.
Table of contents
1. Introduction
2. Confidence intervals
3. Effect size
4. Cohen Effect size for mean difference
5. Effect size for non normal - data
6. Conclusions
1. Introduction
Computational statistics along with statistical software packages can describe and shape any biomedical phenomena using bootstrap replication methods [1]. With the help of these bootstrap techniques, it is possible to estimate using confidence intervals any statistical parameter belonging to a statistical population which does not respect the hypotheses of classical inferential statistics.
Challenges occur when data distribution is uncertain and fails to be approximated with a normal one and, last but not least, when we deal with insufficient number of data, thus not being able to obtain a known statistical distribution of the data at a reasonable risk. The hypotheses of inferential statistics require a selection volume of at least 30 values, which disagrees with the ethical code and the economic conditions of animal experiment studies [2]. Thus, the research on the number of animals necessary to obtain credible results is pervasive [3] but, also, the statistical approach must be modern, based on computational simulations [4].
The bootstrapping technique, also called resampling, is based on random replications having sample extractions returns (that is, the same individual may be encountered several times in the bootstrap set and also in any other bootstrap sample), from the dataset under analysis, replications having the same size as the original dataset. This method assumes that the original dataset is randomlyextracted from an infinite population and is representative of it.
This bootstrap resampling technique is also used in bioequivalence studies of formulations to obtain a confidence interval for the difference of two treatments, one tested and one reference [5].
This article is divided into four sections. In the first section, we analyze the need to use computational techniques when the hypotheses of inferential parametric statistics are not accomplished. The second section describes several models of bootstrap confidence intervals and the assumptions they imply. In the next section, we amplify the new statistics approach by exposing the effect size measures both under normality conditions and when this assumption is not met. The last section is reserved for conclusions.
Click here see the full version.
Contributo selezionato da Filodiritto tra quelli pubblicati nei Proceedings “17th Romanian National Congress of Pharmacy - 2018”
Per acquistare i Proceedings clicca qui:
https://www.filodiritto.com/proceedings
Contribution selected by Filodiritto among those published in the Proceedings “17th Romanian National Congress of Pharmacy - 2018”
To buy the Proceedings click here: